AI-generated art found its glory first with the Portrait of Edmond de Belamy auction in 2018, which sold for a whopping $350,000.
AI art’s popularity has been nothing short of an epic story since then. With AI models like DALL E, Stable Diffusion, and Midjourney, AI art has become more than just a trend empowering business activities.
AI has become so powerful that it needs just 1/4th of an image or just a few words to generate new images.
AI-generated art helps businesses create unique and visually appealing marketing content, graphics, and personalized resources to enhance their brand experience.
Though there are no limits to what you can do with AI art generation, understanding how it works makes the creative process much more efficient. This article focuses on how AI art works, its models, use cases, and more.
What is an AI art?
AI art, also known as artificial intelligence art, encompasses various forms of digital artwork that are either generated or enhanced using AI tools.
How AI art works?
AI art involves using machine learning algorithms to generate or modify art images. The specific workings of AI art vary depending on the model employed. For instance, Generative Adversarial Networks (GANs) are commonly used in AI art and rely on deep learning techniques such as convolutional neural networks to create generative image samples.
Generative modeling, a task within machine learning, is an unsupervised learning approach where algorithms autonomously discover and learn patterns in input data. Once trained on these data patterns, the model can generate new samples that resemble the original dataset.
The GAN model is at the heart of generative AI art
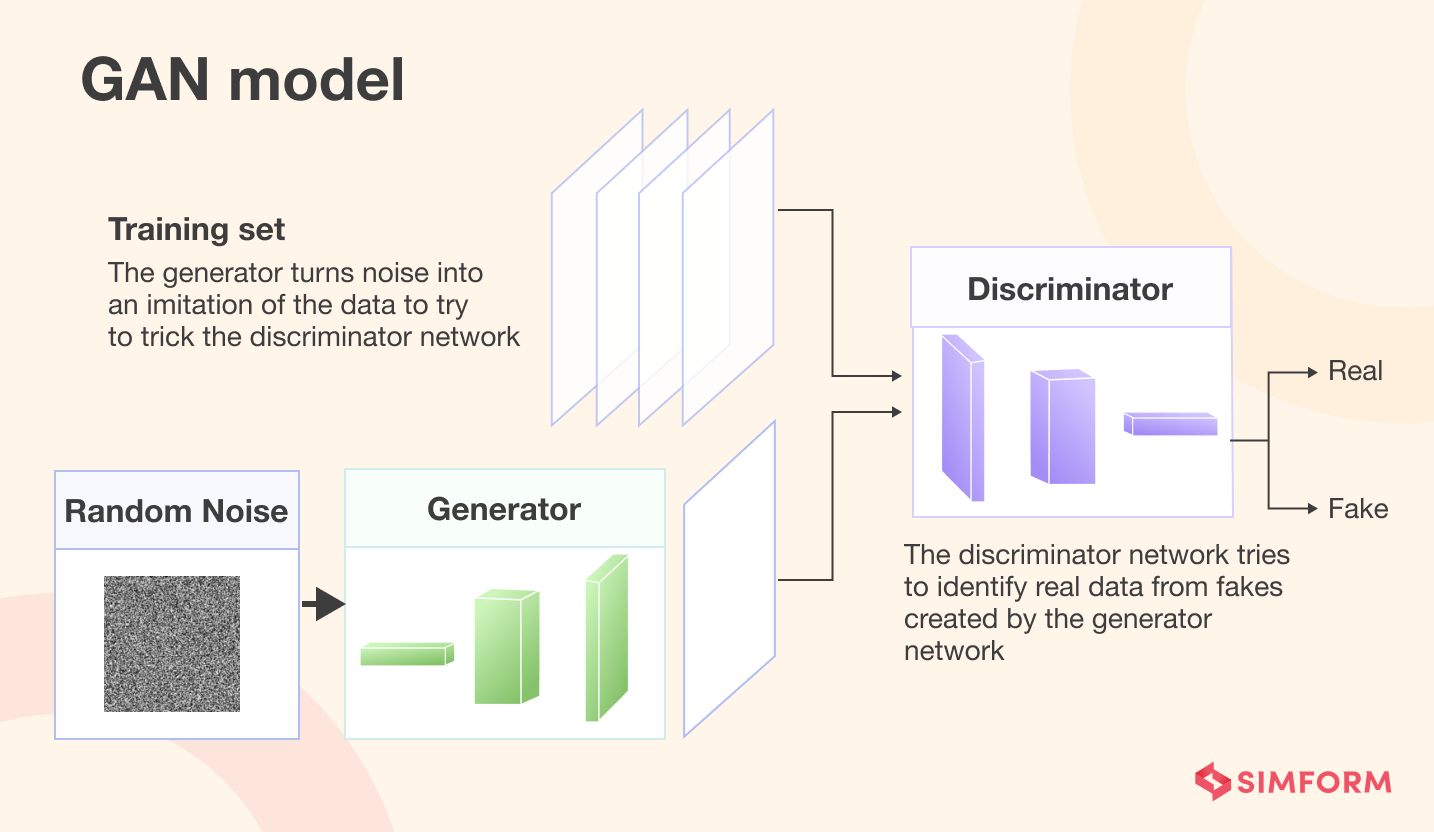
GANs form the core of generative AI art. These powerful machine-learning models have revolutionized the field by enabling the creation of new and original artworks.
The model uses two major components, the generator, and the discriminator, that work together competitively. The generator produces synthetic art samples based on random noise and learns from the training data to improve its output quality. Meanwhile, the discriminator acts as a critic, distinguishing between real and generated art samples.
During training, the generator and discriminator compete against each other. The generator aims to deceive the discriminator, while the discriminator seeks to identify real samples accurately. Through iterations of training, both components enhance their performance.
Over time, the generator generates artwork that resembles reality, while the discriminator becomes more skilled at differentiating real and generated art. This iterative process produces high-quality generative AI art, as the generator learns to fool the discriminator and achieve realistic and artistically impressive results.
Generative AI art tools built on GANs
- IBM’s GAN Toolkit uses GAN models to provide a highly adaptable no-code solution to facilitate the creation of generative AI art.
- HyperGANis a modular GAN framework with an API and user interface that helps businesses build custom models to generate AI art.
- GAN Labis a visual, interactive experimentation tool that provides a drag-and-drop interface for building and training GANs, which can be used for building art generation tools.
- Midjourney uses GANs and progressive growing to generate images that look like actual photographs.
- StyleGAN is a type of GAN that helps generate high-quality images with realistic fixtures and details in image synthesis.
- CycleGANis a tool that uses GANs for image-to-image translation and converts one style to another.
- DALL-Euses GANs to convert text prompts into images.
- GauGAN is a tool developed by Nvidia that uses GANs to generate realistic landscapes from simple sketches
Though GAN models are at the core of many AI art generation tools, other models help create AI-assisted art. Let’s understand some of these AI models in brief.
Other AI art models
Several common generative AI art models have gained popularity due to their remarkable capabilities. These models provide the underlying technology which facilitates AI art generation for artists, professionals, and businesses. Some notable AI art models include:
Stable Diffusion
Stable Diffusion is a text-to-image model allowing AI generator tools to generate images based on user prompts. It uses pre-trained weights, models, or checkpoint files to generate images based on the provided input.
The types of images generated depend on the data used to train the model. For example, a model trained on cat images will predominantly generate cat images.
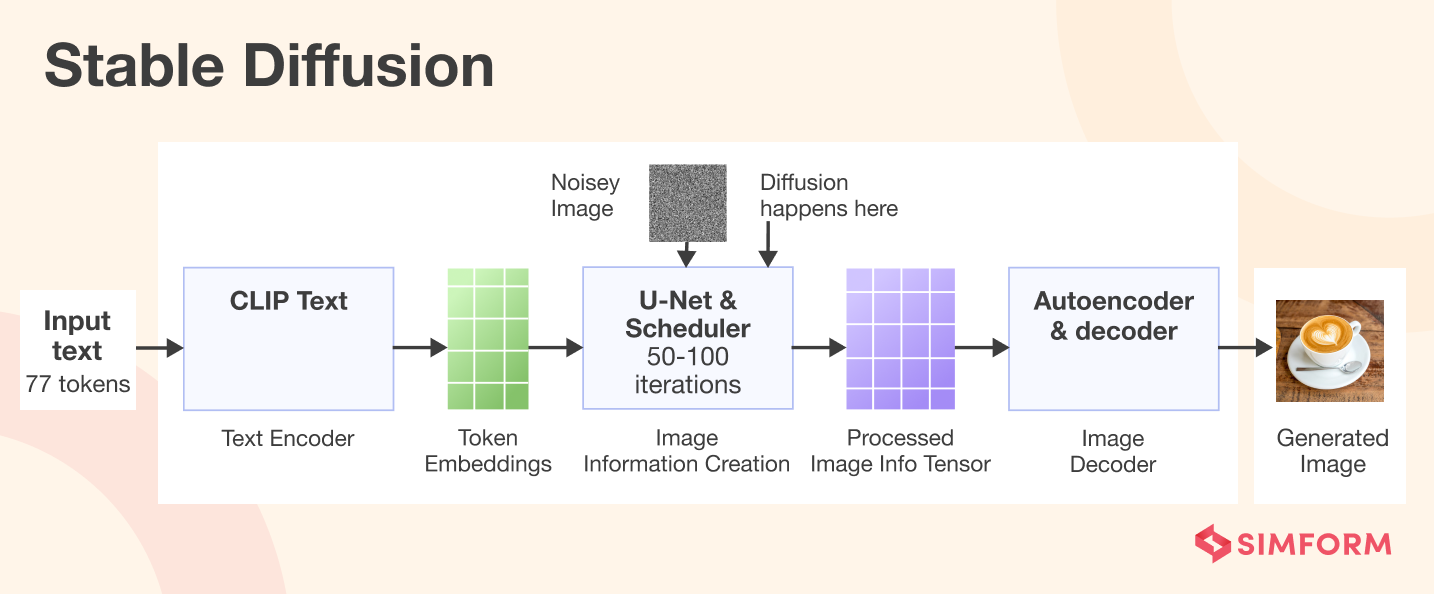
Stable Diffusion involves learning the underlying patterns in a dataset and encoding them in a latent space. This latent space is like a condensed area where the image information is stored. The model uses an attention mechanism that combines input and conditioning images to generate noise.
The key element of the diffusion model is noise. It adds noise to the image in a progressive manner during training and removes details. Eventually, the image turns into pure noise, and then the model begins to denoise and recreate the image.
The Stable Diffusion tool with a text prompt starts with a randomly generated noisy image in the latent space. The model continues to denoise the image for a set number of steps, usually between 50 to 100 until it produces AI art in a 512×512 image format that is easily visible to humans.
In essence, generating art in Stable Diffusion is like unmixing a sugar cube dissolved in hot milk.
Generative AI art tools that use Stable Diffusion
- Dream Studiois an innovative tool that uses Stable Diffusion to transform text prompts into visually captivating artworks.
- DiffusionBee is another exciting tool that uses Stable Diffusion to generate art with an intuitive interface and various parameters to tweak.
DALL E
DALL-E is a significant large language model (LLM) developed by OpenAI that can generate images from text descriptions. It was trained on a massive dataset of text and images and can now create realistic and detailed images from various prompts.
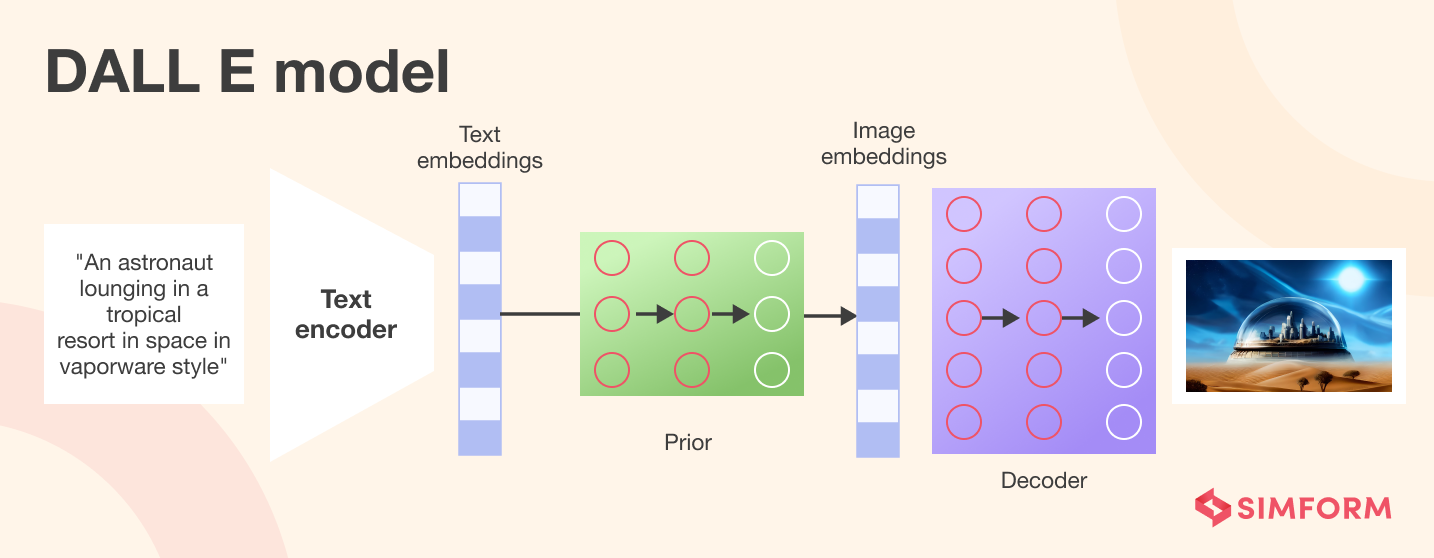
When a text prompt is given, a text encoder creates text embeddings. These embeddings are then input for the Prior model, which generates image embeddings. An image decoder model is then used to generate the final image from these embeddings.
DALL E is an AI model and a platform for artists and designers to generate art. Recently OpenAI introduced a new version of DALL E called DALL E 2, which uses a CLIP model.
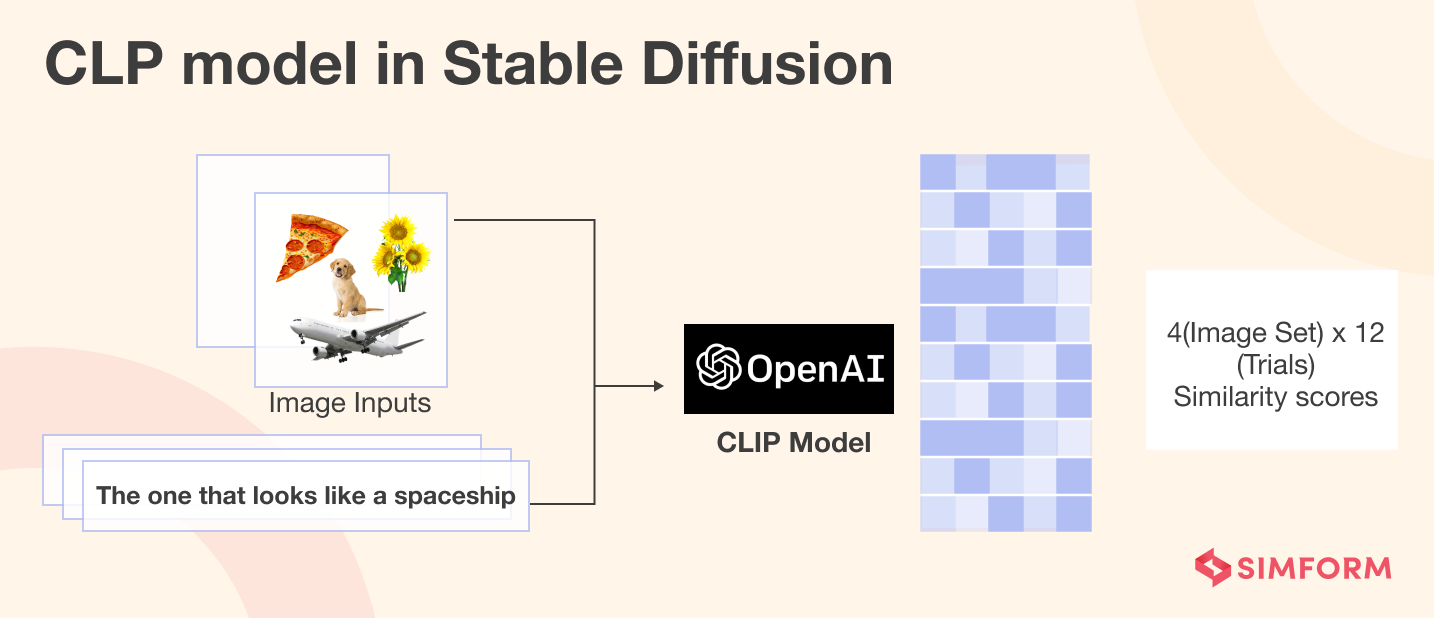
A neural network model called CLIP (Contrastive Language-Image Pre-training) finds the most appropriate caption for a given image. It performs the inverse of what text-to-picture generation in DALLE 2 does.
To learn the relationship between the textual and visual representations of the same thing, CLIP has a contrastive purpose as opposed to a predictive one, like predicting or categorizing a picture.
Generative AI art tools that use DALL E
- Cubism is a web-based tool that uses DALL-E to generate images from text descriptions.
- Dream by Wombo is an AI-powered platform that uses DALL-E to create 3D animations from text descriptions.
Imagen
The Imagen AI model by Google is a text-to-image diffusion model that aims to generate realistic images based on textual descriptions. It leverages large pre-trained frozen text encoders and diffusion models to achieve high photorealism and a deep language understanding.
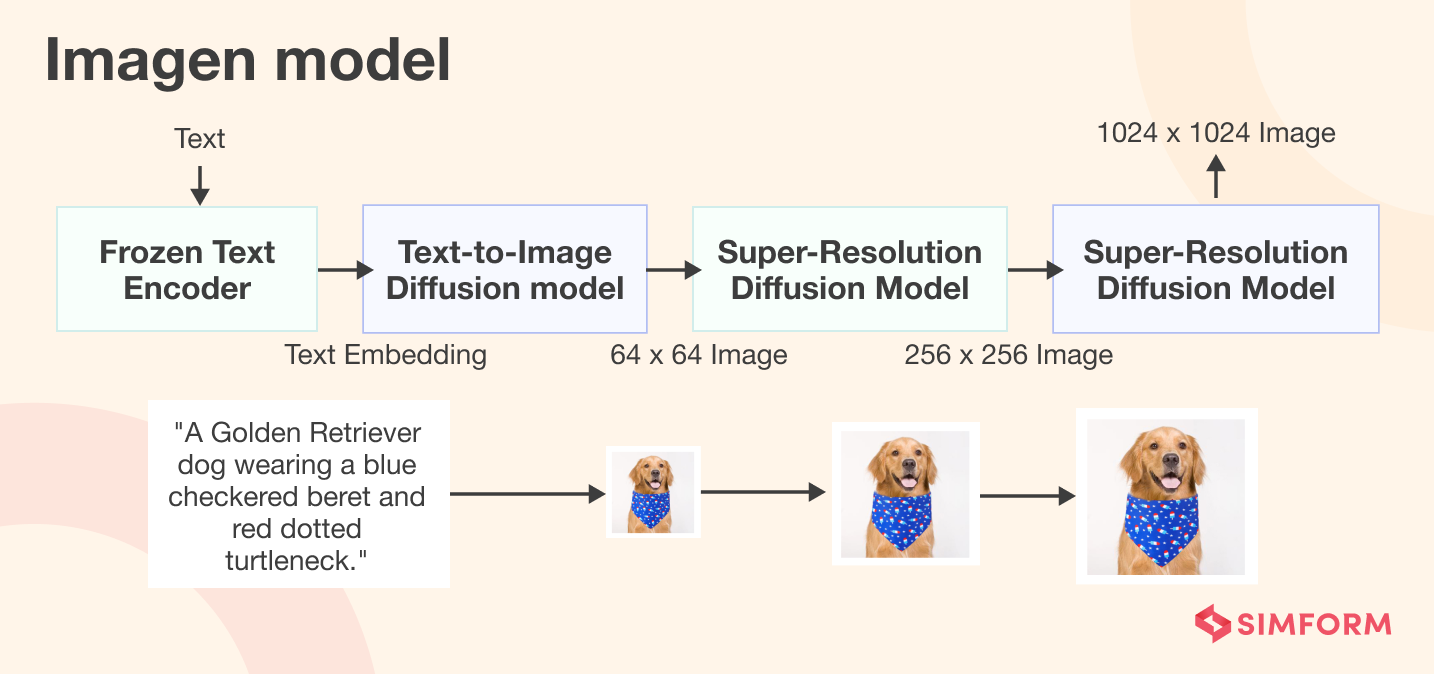
The Imagen model demonstrates the effectiveness of diffusion models for text-to-image generation, surpassing the performance of autoregressive models, GANs, and Transformer-based methods.
Imagen uses larger pre-trained frozen language models, which contribute to improved image fidelity and image-text alignment compared to other models that use cascaded diffusion models or BERT as a text encoder.
Imagen is part of a series of text-to-image work at Google Research and its sibling model Parti. However, this model is still under development by Google and will soon disrupt the AI art generation market.
Midjourney
The MidJourney AI model for art generation is a deep learning model designed to generate new and original paintings. This model was created by Turkish artist Refik Anadol and his team, using diffusion models to generate art.
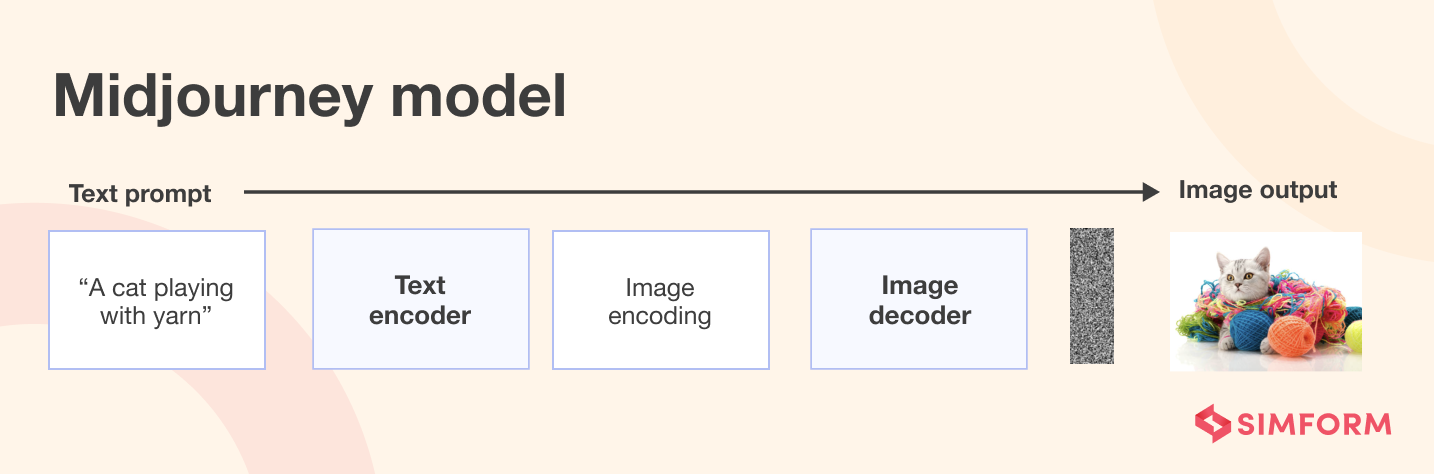
The MidJourney AI model works by feeding a large dataset of images into a deep learning network trained to extract the visual features and patterns in the images. The model then uses this knowledge to generate new images with similar visual characteristics.
One unique aspect of the MidJourney AI model is that it allows for user interaction and input. Users can upload their images, which the model will analyze and use to generate new artwork that is a fusion of the original image and the learned visual features.
The output images from the MidJourney AI model are often abstract and surreal, as the model is not limited to creating representational art. This makes it a helpful tool for artists and designers looking to create unique and visually striking works.
Deep Dream Generator
The Deep Dream Generator AI model is a neural network implementation that can generate creative images with unique features from different input images. The model was first introduced by Google in 2015 as a way to visualize the patterns and features learned by a convolutional neural network (CNN).
The basic idea behind Deep Dream is to use a pre-trained CNN, such as Google’s Inception V3, to generate an image that activates specific neurons in the network. This is done by iteratively modifying an input image to amplify the patterns and features that the network has learned.
The process starts with a base image, an image the user provides, or a random noise image. The base image is fed into the pre-trained CNN, and specific neurons’ activations are identified. These neurons represent features that the network has learned, such as edges, textures, or objects.
We can enhance the base image by emphasizing the patterns and features identified by specific neurons. This is achieved by analyzing and adjusting their gradients through a process called backpropagation. By doing this, we gain insights into how these neurons play a role in the overall functionality of the model.
The process is repeated multiple times, with the modified image from the previous iteration being used as the base image for the next iteration. This creates a sequence of images that progressively enhance the patterns and features in the input image. It results in a new image as output which is realistic and close to the original data source.
With tools like TensorFlow 2.0, it is now easier than ever for developers and enthusiasts to experiment with this fascinating technique.
AI art Examples
There are several examples of generative AI art that you can find on the internet. However, here are some which have been widespread, beginning with the first one from the legendary Sam Altman- the pioneer of generative AI
DALL·E 2 is here! It can generate images from text, like “teddy bears working on new AI research on the moon in the 1980s”.
It’s so fun, and sometimes beautiful.https://t.co/XZmh6WkMAS pic.twitter.com/3zOu30IqCZ
— Sam Altman (@sama) April 6, 2022
Another example of the AI art generated on Midjourney by a user known as @Agua sho+wcases how an AI-based image is generated from a basic text prompt.
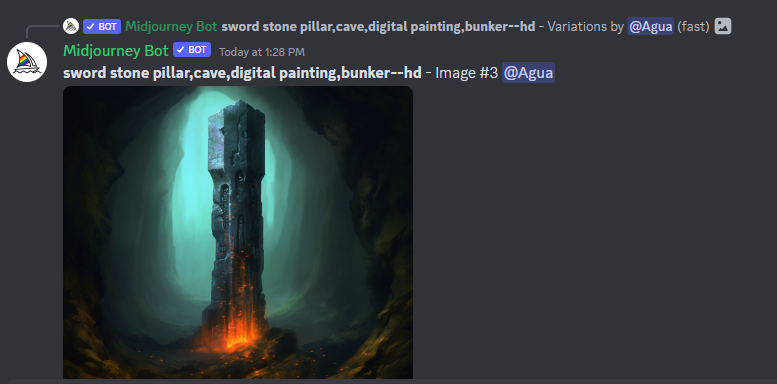
Digital art for New York City of 2088 is also an interesting AI artwork created using DreamStudio with Stable Diffusion at its core.
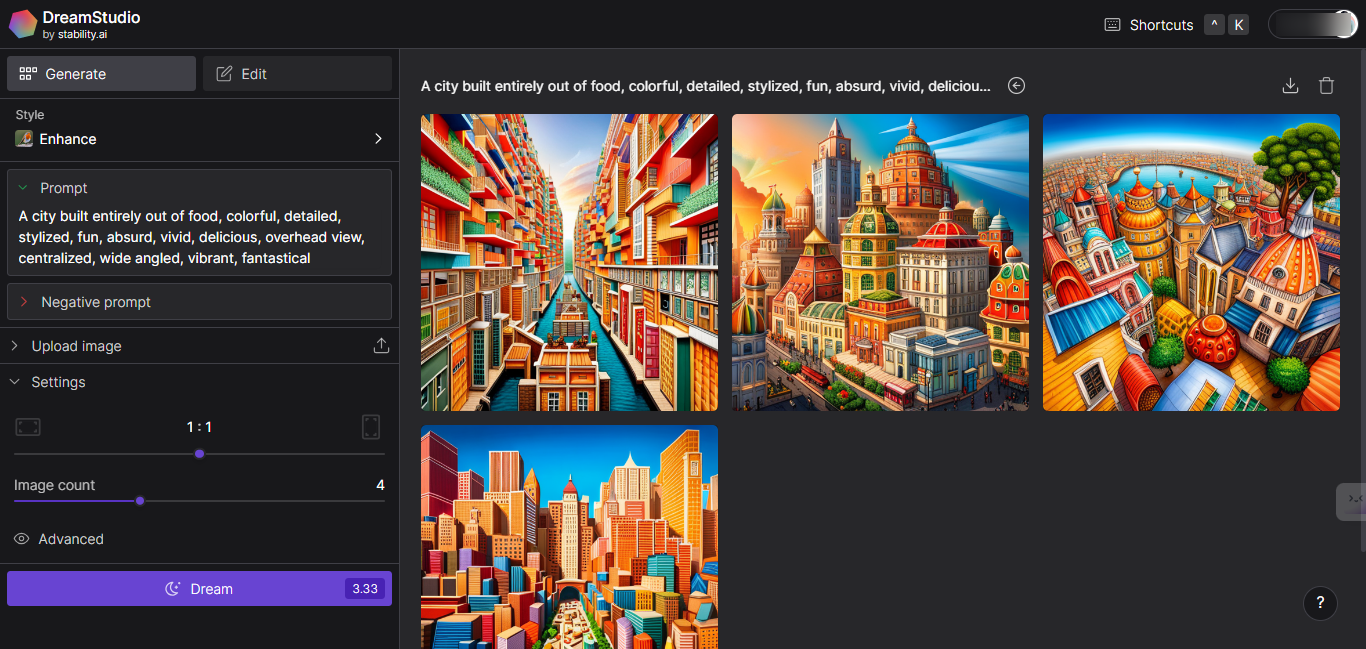
AI art generation use cases
- Concept Art: AI-generated art can quickly produce a range of concept art for various purposes, such as video games, movies, or design references. Artists and designers can instantly explore their imagination and generate multiple concepts and styles, saving time and resources.
- Digital Art: AI art generators enable artists to create intricate and detailed artwork in a less time-consuming manner. Artists can use AI to generate many potential designs, which they can modify and refine to create their final artwork.
- Comic Books or Graphic Novels:AI-generated art can be used to create comic books or graphic novels. Artists can leverage AI to generate backgrounds, characters, and other visual elements, speeding up production.
- Logos and Icons: AI art generators can assist in the creation of logos and icons. Designers can quickly generate various options and refine them based on their requirements.
- Enriching Personal Photos and Portraits: Generative AI art can enhance personal photos and portraits. Using AI algorithms, images can be retouched, augmented, or transformed into artistic styles, creating unique visual effects.
- Photo Editing and Manipulation:AI-powered tools can facilitate photo editing and manipulation. They can automatically enhance images, remove unwanted objects, or apply artistic filters to achieve desired effects.
- Cover Art Design:Generative AI art can contribute to cover art design for various media, such as books, albums, or magazines. It allows designers to explore different visual concepts and create eye-catching covers quickly.
- Design UX/UI: AI-generated art can be employed in user experience (UX) and user interface (UI) design. It can assist in creating visually appealing interfaces, generating icon sets, or suggesting design elements based on user requirements.
- Promotional and Marketing Assets: AI art generation can support the creation of promotional materials and marketing assets. It enables designers to generate compelling visuals, illustrations, or animations for advertising campaigns or branding purposes.
- Fashion Design: AI-generated art can be used in fashion design to explore new patterns, textures, or color combinations. Designers can generate unique designs and experiment with various styles and
The rise of AI in art
AI art is artwork, visual, audio, or otherwise, created or enhanced using AI tools. However, visual AI is not new to businesses; tech giants like Apple and Google have been working on AI-based image curation within their photo apps.
What has changed in the last few years is the introduction of generative AI capabilities for art creation. You can just input a few words as a prompt, and the generative AI art tool will create art stunning artwork for you in an instant.
Hollywood studios now use generative AI art to create animations for entire movies, like “Spider-Man: Into the Spider-Verse.”
Here is a short timeline of how AI art generation evolved over the years!
- 1960s: The first AI art generators are developed. These early generators are very simple and can only create basic geometric shapes.
- 1970s: Generative AI art tools became more sophisticated and could create more complex images, such as landscapes and portraits.
- 1980s: AI art generators are used to create commercial art, such as advertising and video game graphics.
- 1990s: Generative AI tools became more accessible to the general public and are used to create personal art projects.
- 2000s: AI tools helped create realistic images indistinguishable from human-made art.
- 2010s: Generative AI tools can now create new art forms, such as generative and algorithmic art.
- 2020s: AI art generators create interactive and immersive art.
- 2022: Midjourney, Stable Diffusion, and DALL-E 2 are released. These new-age AI art generators can create stunningly realistic and creative images.
- 2023: AI art generators continue to evolve, becoming more powerful and versatile. They are used to create art for various purposes, including commercial, personal, and artistic expression.
Impact of Dall E & Midjourney on the Art Industry
DALL-E and Midjourney leverage AI algorithms to generate images, creating AI art and contributing to a rapidly growing area of AI-generated art. DALL-E specifically marked a turning point in generative AI art with its ability to produce detailed and realistic images.
Here is a simple example of how DALL E revolutionizes the process and allows you to create stunning art with textual prompts.
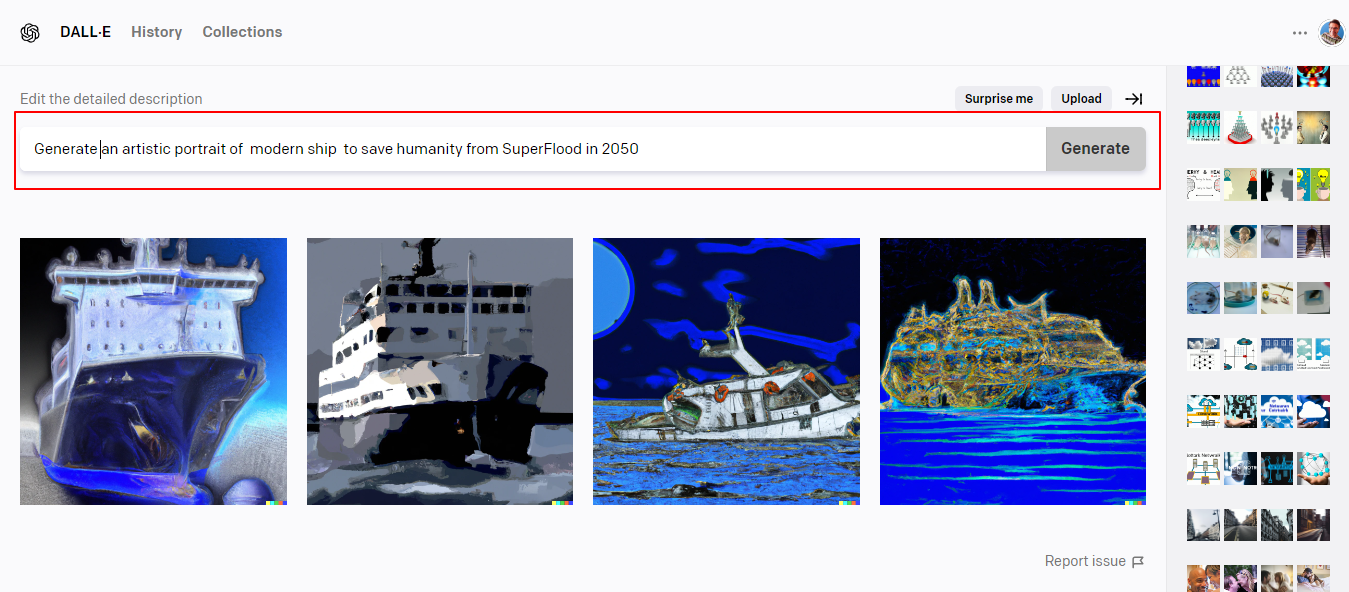
AI tools like DALL E and Midjourney empower professional artists and casual users to create visually appealing images easily. Users can instantly generate multiple variations of AI artwork and quickly iterate on ideas without technical expertise.
🙏Thanks to @icreatelife for the inspiration to create this prompt for creating incredible luminescent scenes (check the ALTs).
I would love to see your creations! 🏜️✍️#AIArt #Midjourney pic.twitter.com/UTcSujXsoq
— TechHalla (@techhalla) June 7, 2023
The impact of these generative AI models goes beyond aesthetics, as the realistic textures produced by tools like DALL-E have significant potential in fields such as game development, 3D modeling, and virtual reality. These models can enhance games by providing realistic graphics that create a more immersive experience for players.
Additionally, artists and designers can use Stable Diffusion models (explained in the latter sections) with a Blender to create new texture-based 3D designs. Such use cases make generative AI art far more attractive for businesses as it saves time and offers creative designs.
It’s not surprising that AI-generated art has also found a place in the world of Non-Fungible Tokens (NFTs), where artists use generative AI art as NFTs, selling them to collectors and generating high value in the process. This intersection between AI-generated art and NFTs provides exciting opportunities for artists to showcase and monetize their creations.
The project La Collection is an example of a digital twin of physical art, bridging the gap between the two mediums.
How are contemporary artists using AI art?
Contemporary artists are embracing AI art to explore new creative possibilities and push the boundaries of artistic expression. They use AI tools and algorithms to enhance their artistic process in various ways. Some of the common approaches include:
Creating art with human-machine Collaboration
With the advent of artificial intelligence (AI) in the art world, artists now have the opportunity to collaborate with AI systems to create artwork.
As a part of this collaboration, artists train AI systems on their own artistic styles and techniques. This allows the AI to generate unique artworks that reflect the artist’s vision, blurring the lines between human and machine creativity.
Augmenting processes
Artists also leverage AI algorithms to generate initial ideas, create complex patterns or textures, or experiment with different compositions.
Exploring algorithms
Artists are delving into the algorithms themselves, experimenting with AI models and tweaking their parameters to create novel visual effects. They are using the generative capabilities of AI to generate new and unexpected forms, textures, and patterns.
Getting feedback on artwork
Through image recognition and pattern recognition algorithms, AI systems can evaluate and analyze different aspects of an artwork, such as composition, color usage, and style. This feedback can be valuable for artists seeking to refine their artistic techniques or understand how others perceive their work.
Creating large quantities of art
Through machine learning algorithms, AI systems can learn from existing artworks and create new pieces based on learned patterns and styles. This ability to generate art in large quantities can benefit various purposes, such as creating art for commercial purposes, exploring different artistic variations, or experimenting with artistic concepts.
Exploring digital art market opportunities
Digitalizing the art market has created new opportunities for artists to showcase and sell their work. AI plays a role in this context by enabling artists to leverage digital platforms, online auctions, and virtual art-viewing technologies to reach a broader audience.
By embracing digital tools and platforms, artists can tap into the growing digital art market, connect with potential buyers, and explore new avenues for artistic expression and commercial success.
Leveraging generative AI art: A brave new world!
Art needs creativity, and it does take time to create artistic elegance, but what AI has been able to do is make this process rapid. This is especially important for businesses that need graphics, art, and UI/UX designs faster for a competitive edge.
Designing these graphics with AI offers faster iterations making A/B testing of designs easier for organizations. However, understanding the architecture is key if you want to integrate these AI art models. We have discussed some of the popular AI art generation models, with some of them providing text-to-image generative capabilities.
If you want to know more about different generative AI models and their use cases, here is an excellent article. Contact us for more information on AI integrations and use cases.