From playing music to making reservations, virtual assistants like Alexa and Siri can do it all. Ever wondered how they understand what we want? How do they manage to find the right answer so quickly? That’s the power of natural language processing at work!
This cutting-edge technology allows virtual assistants to analyze spoken and written language, precisely deciphering meaning and intent. What’s more, with each interaction, these digital helpers get even smarter, making our lives easier and more connected than ever before.
And this is not the only NLP use cases! The potential applications of NLP are virtually limitless and can fundamentally revolutionize many industries by automating complex tasks, optimizing operational efficiency, and providing invaluable insights.
From healthcare to eCommerce, finance to legal services, NLP techniques are shaping the future of businesses and creating new opportunities for growth. This article will provide a comprehensive overview of NLP applications across various industries and highlight their potential impact.
What is Natural Language Processing (NLP)?
Natural Language Processing (NLP) empowers computers to understand, interpret, and generate human language. You encounter NLP daily, often without realizing it. You interact with NLP technology when you use voice assistants, translate text online, or receive auto-generated email responses.
At its core, NLP bridges the gap between human communication and computer understanding. It leverages artificial intelligence to break down language into smaller, analyzable components, allowing machines to grasp the context, sentiment, and intent behind words.
NLP has two main components: natural language understanding and natural language generation. The understanding aspect involves teaching computers to comprehend human input, while the generation part focuses on producing human-like responses.
What are the key components of NLP utilized in various business applications?
Two key components of NLP – Natural Language Understanding (NLU) and Natural Language Generation (NLG) are reshaping how businesses handle language-based tasks..
NLU interprets human language input, and extracts meaning and intent. NLG produces human-readable text from data. These technologies power applications like chatbots, automated reporting, and personalized content creation.
Natural Language Understanding (NLU)
- Interprets the meaning of text or speech by analyzing its syntactic structure and semantic content.
- Identifies and categorizes key entities such as names, dates, and locations within a given text.
- Determines the sentiment expressed within a text or speech, whether positive, negative, or neutral.
Natural Language Generation (NLG)
- Involves organizing and structuring content logically before generating human-readable text for the coherent, relevant, and effective conveyance of the intended message.
- Produces text using predefined templates or advanced techniques, enabling businesses to generate content tailored to specific needs, from simple responses to complex, dynamic narratives.
- Condenses large volumes of information into concise summaries while preserving the core message, benefiting industries dealing with vast amounts of data, such as legal services, finance, and research.
By understanding and leveraging the components of NLP, you can unlock the potential of generative AI in diverse applications and develop innovative solutions.
Natural Language Processing applications:
NLP for businesses
Before delving into the specific natural language processing applications across various industries, it’s worth exploring some of the most common yet innovative ways NLP is being used to revolutionize the way we communicate and interact with technology.
Text classification
Text classification involves categorizing unstructured text into predefined categories using rule-based systems or machine learning. Text classifiers assign relevant tags to content, aiding in efficient document management and extracting valuable insights.
A prime example is LinkedIn, which employs text classification to flag profiles containing inappropriate content. Machine-based classifiers often use the “bag of words” representation, which simplifies text by focusing on word frequency to provide a clear understanding of the content’s context.
Text extraction
Text extraction utilizes machine learning to automatically identify and extract relevant words, phrases, and specific information from unstructured text, such as articles, surveys, and customer service tickets.
This process, also known as keyword extraction, offers various NLP benefits, including summarizing texts, cleaning data, and uncovering common or recurring themes.
For instance, a text extractor can analyze a statement like, “While the new design is visually appealing, the cost is rather high and the speed is impressive,” to extract keywords such as “visually appealing,” “cost,” and “speed,” providing valuable insights for decision-making.
Text extraction simplifies large amounts of data by identifying key phrases and words efficiently.
Text summarization
Text summarization simplifies large volumes of data by extracting relevant information, making it easier for users to access vital points. It works through extraction-based methods, selecting key phrases, or paraphrasing the original content.
A unique example of text summarization is the automatic generation of news headlines, providing readers with a concise understanding of the article without delving into every detail.
Machine translation
Machine translation (MT) translates text between languages using machine learning algorithms and neural networks to process linguistic data.
MT provides numerous benefits, especially in business applications and social media platforms, by enabling seamless communication across different languages. For example, Facebook and Instagram integrate MT in their applications, connecting users globally. Despite challenges in handling idioms and ambiguity, MT has made significant progress in recent years, offering fast and cost-effective translations.
Sentiment analysis
Sentiment analysis automatically interprets and summarizes emotions within textual data. Using machine learning algorithms, it can detect emotions such as “positive,” “negative,” or “neutral,” along with more specific sentiments like “anger,” “joy,” or “disgust.” Sentiment analysis is invaluable for businesses to gauge customer opinions on brands, products, and services based on online conversations or direct feedback.
Conversational agents
Conversational agents, such as chatbots and virtual assistants like Siri, Alexa, and Google Assistant, facilitate seamless communication between humans and computers via text or speech. These agents work by understanding user commands, processing the language, and providing appropriate responses.
We’ve discussed the most well-known natural language processing examples, but it is also being used in a variety of other useful contexts, including market intelligence, auto-correct and auto-prediction, grammar checkers, email classification and filtering, speech recognition, intent classification, urgency detection, etc. Also, don’t get confused between NLP and NLU. Now, let’s understand how these applications are used across various industries.
NLP in eCommerce and retail
The eCommerce and retail landscape is fiercely competitive, necessitating businesses to stay ahead of the curve. By leveraging NLP, companies can gain valuable insights into customer preferences and behavior, ultimately driving sales and customer satisfaction.
Product recommendation systems
NLP can be used to analyze customer preferences and purchase history to provide personalized product suggestions. For example, an online clothing store could recommend a specific style or brand based on a customer’s previous purchases and browsing patterns.
Sentiment analysis
By analyzing customer reviews and feedback, NLP can help businesses identify trends and areas for improvement. This information can be used to refine product offerings, improve marketing strategies, and enhance the overall customer experience.
Personalized marketing campaigns
NLP can help create targeted marketing campaigns by analyzing customers’ preferences, interests, and online behavior. For instance, a travel agency could send tailored email promotions to customers interested in specific travel styles or destinations.
NLP in healthcare: Applications and opportunities
The healthcare industry is constantly evolving, and NLP has a significant role to play in its transformation. By automating processes and providing valuable insights, medical professionals can make informed decisions and improve patient care.
Medical transcription
NLP can convert spoken language during doctor-patient interactions into written documentation, saving time and ensuring accurate patient records.
For example, a speech-to-text system could transcribe a doctor’s verbal notes about a patient’s symptoms and treatment plans.
Clinical documentation improvement
NLP can streamline and enhance the process of maintaining accurate and comprehensive patient records. By analyzing clinical notes, NLP algorithms can identify gaps or inconsistencies in the documentation, ensuring that healthcare providers have a complete picture of a patient’s medical history.
Patient support through virtual assistants
NLP-powered virtual assistants can drastically improve patient engagement and satisfaction rates by answering questions and providing personalized support. For instance, a chatbot could help patients schedule appointments, remind them to take medications, or provide information about their condition.
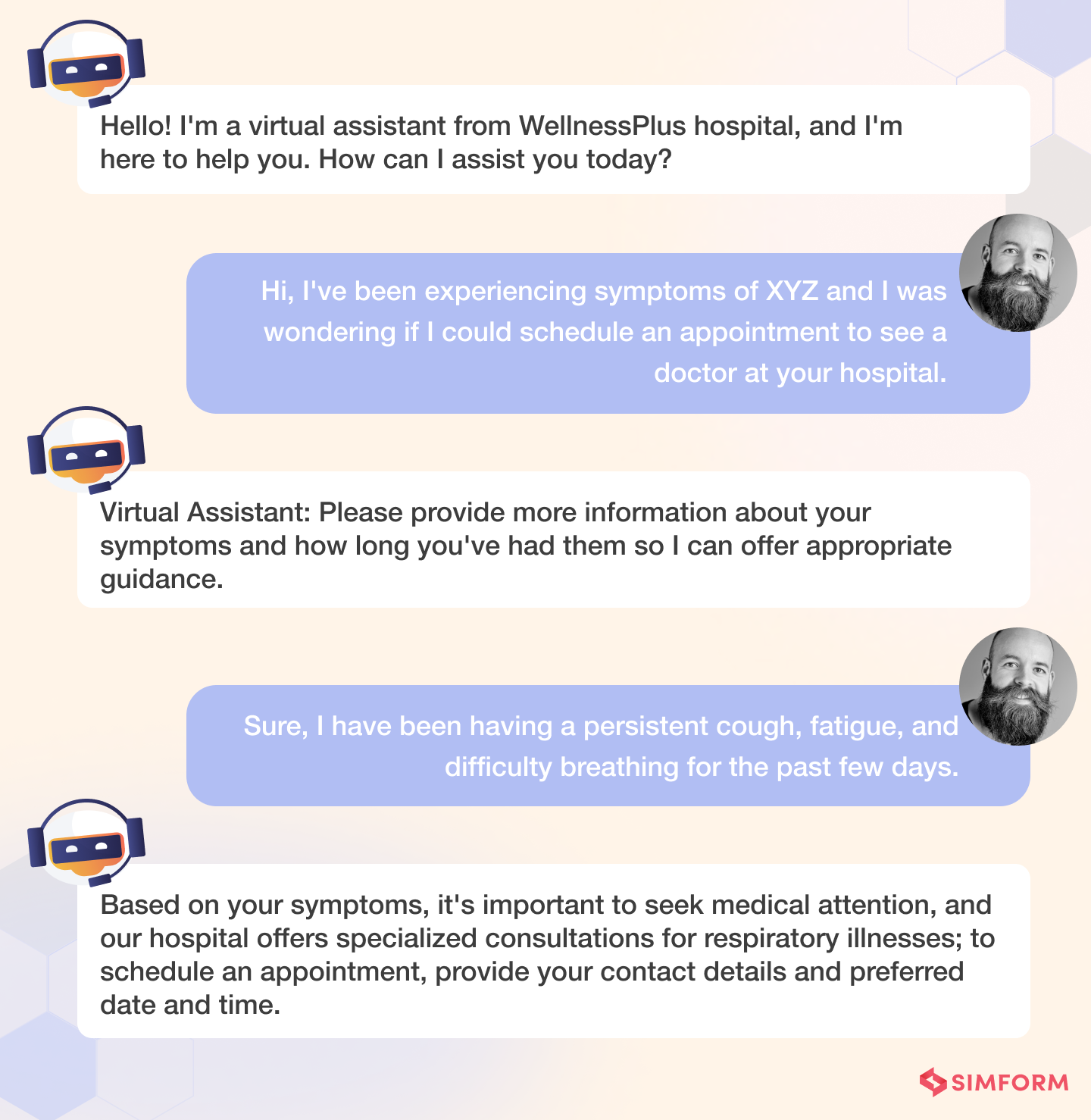
NLP in finance
In finance, the ability to process and analyze large volumes of data quickly and accurately is essential. NLP can be a valuable asset by providing insights, automating tasks, and improving the customer experience.
Sentiment analysis
By analyzing news articles, social media posts, and other textual data, NLP can help financial institutions gauge public opinion about stocks, bonds, or other financial instruments. This information enables them to make more informed investment decisions and potentially predict market trends.
Fraud detection and prevention
NLP’s text analysis capabilities can help pinpoint suspicious patterns in communication, such as emails, text messages, or transaction records, to identify potential fraud. For example, an algorithm could detect unusual language in an email that might indicate a phishing attempt.
Chatbots for customer support
NLP-powered chatbots can greatly enhance the customer experience by providing instant assistance and reducing wait times. These virtual assistants can answer common questions, assist with account management tasks, and even guide customers through complex financial transactions.
Other than the above use cases, NLP is also useful in various other aspects of finance, such as
- Loan risk assessment: Analyzing borrower’s data to predict the likelihood of default and determining credit worthiness.
- Auditing and accounting: Automating the analysis of financial documents, identifying discrepancies, and ensuring regulatory compliance.
- Portfolio selection: Assisting investors in making informed decisions by analyzing market sentiments, news, and trends.
- Insurance: Enhancing underwriting processes, claims management, and fraud detection through text analysis.
NLP in customer service
When it comes to customer service, NLP truly shines. By automating basic tasks and providing instant support, NLP can free up human agents to focus on more complex issues, ultimately improving customer satisfaction and loyalty.
Chatbots and virtual assistants
NLP-powered chatbots and virtual assistants can handle a wide range of customer inquiries, from answering frequently asked questions to assisting with account management tasks. By providing instant support, these AI-powered tools can reduce wait times and improve the overall customer experience.
Sentiment analysis and emotion detection
NLP can analyze customer feedback, social media posts, and other textual data to detect emotions and sentiment, helping businesses identify pain points and areas for improvement. For example, an analysis of customer reviews could reveal common complaints about a product, allowing the company to address these issues proactively.
Smart call routing
NLP can be used to direct customers to the most appropriate agent or department, reducing wait times and increasing efficiency. By analyzing the content of a customer’s inquiry, an NLP algorithm can determine the best agent to handle the issue, ensuring a smooth and satisfactory experience.
NLP in legal services
Legal professionals can leverage NLP to simplify their workloads and improve efficiency, allowing them to focus on more strategic tasks.
Automated contract analysis
NLP can be used to review contracts and identify potential risks, inconsistencies, or areas of concern. This can save countless hours spent on manual review and help legal teams prioritize their efforts.
Document summarization
NLP algorithms can condense lengthy legal documents into digestible summaries, making it easier for professionals to quickly understand the key points and implications. For example, a lawyer could use NLP to generate a brief summary of a complex court ruling.
Intellectual property management
NLP can be used to identify potential conflicts and monitor infringement cases by analyzing patent applications, trademarks, and other intellectual property filings. This can help legal teams protect their clients’ interests and stay ahead of potential threats.
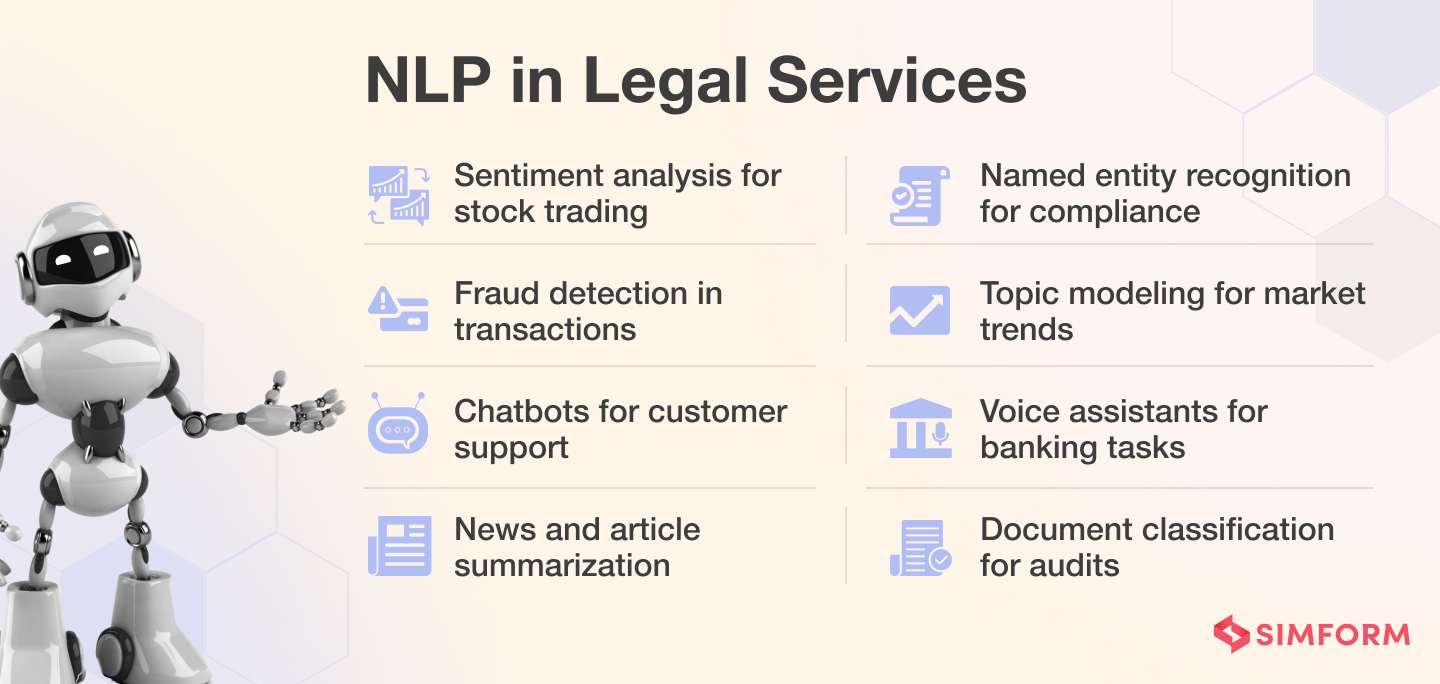
NLP in human resources
Human resources departments can also benefit from applications of natural language processing, as they can streamline processes, save valuable time and resources, and boost employee morale.
Resume parsing and candidate matching
NLP can be used to analyze resumes and match them with relevant job openings, saving recruiters time and effort. For example, an NLP algorithm could identify key skills, experience, and qualifications in a resume and compare them to the requirements of open positions.
Employee sentiment analysis
By analyzing internal communications, employee surveys, and performance reviews, NLP can help identify areas of improvement and boost employee morale. Companies can use this information to address concerns, develop targeted training programs, and foster a positive work environment.
Training and development
NLP can be used to personalize learning experiences and monitor employee progress. For example, an NLP-powered training platform could analyze an employee’s performance data and recommend tailored learning resources to help them develop the necessary skills.
Other than the above use cases, NLP is also useful for:
- Automated interviews: Enhancing the efficiency of the interview process and providing unbiased evaluations.
- Onboarding: Utilizing chatbots to answer employee questions and facilitate a smooth transition into the company.
- Real-time interview feedback: Providing instant feedback during interviews to help candidates understand their performance, creating a more efficient and supportive environment.
- Answers through chatbots: Assisting candidates and employees with quick responses to their queries and concerns.
Drive innovation with applications of NLP
The potential of NLP applications is vast and with the advancements in deep learning and RNNs, it has surpassed human performance in complex tasks, such as machine translation and question-answering NLP systems. Worldwide revenue from the NLP market is forecasted to increase rapidly in the next few years, with the market predicted to be almost 14 times larger in 2025 than it was in 2017.
However, the challenges of domain-specific customization and unpredictable training time and accuracy, call for an experienced partner like Simform to provide customized solutions and expertise to fully capitalize on the potential of NLP applications. .